“My dear, here we must run as fast as we can, just to stay in place. And if you wish to go anywhere you must run twice as fast as that.”
― Lewis Carroll, Alice in Wonderland
Welcome to the first post of Embracing Enigmas! I'll be focusing on what it takes to implement AI/ML well, demystifying hard concepts, and musings on the future.
There's been a lot of buzz this past week from people playing with Open AI's ChatGPT. Some amazing things have been done such as creating code for a SaaS app and then debugging it:
to creating an MBA essay question, then creating the rubric, creating the essay answer to that question, and then scoring it using the rubric:

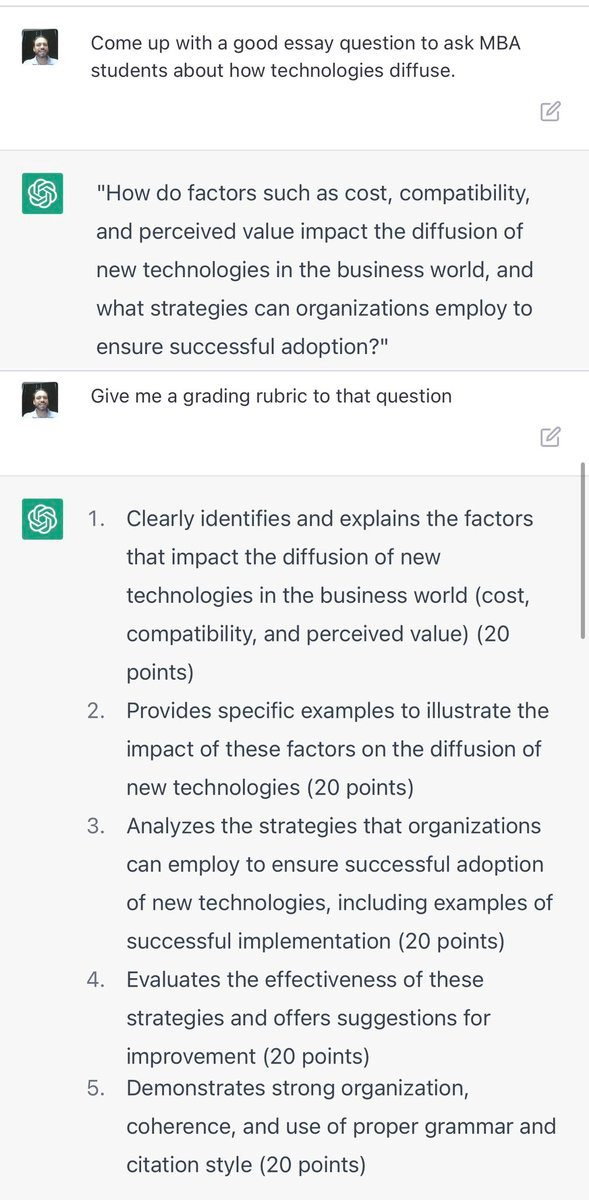
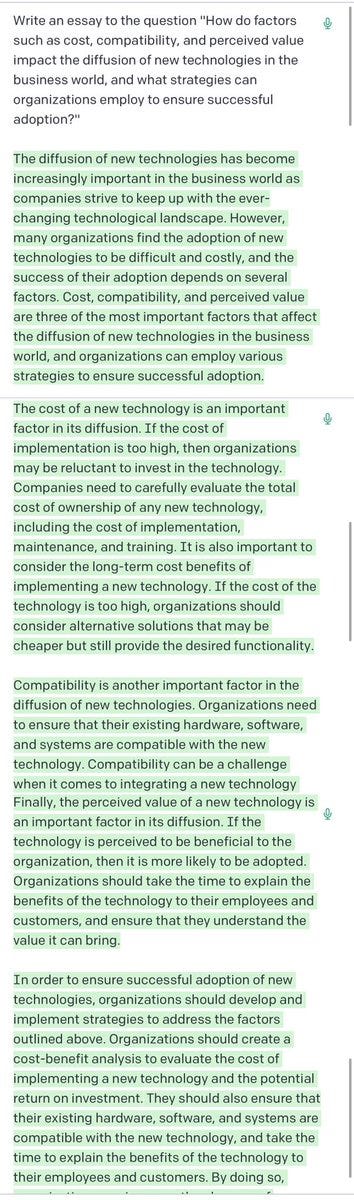
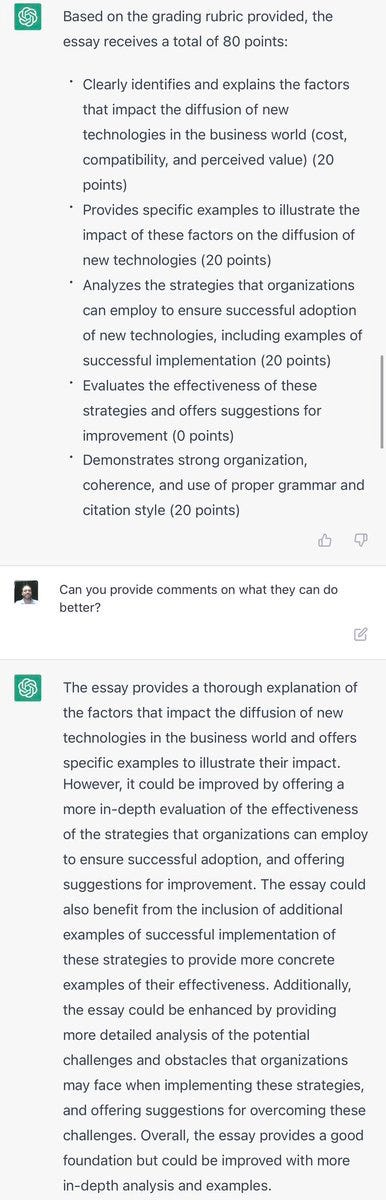
These are some fantastic results! This technology will simultaneously cause a VC hype cycle and a paradigm shift for how we will all engage with the world. While many people have written about all the different uses of this technology, I want to talk about what comes next.
We're about to enter a Cambrian explosion of generative AI startups. Most of these companies will fail because they will miss key elements of what it takes to do generative AI well. A majority of startups will rely on using what's called a foundation model which is a large model that has been pretrained on a very large dataset and can be used for multiple purposes. Typically these models are very costly to retrain in full and the training cost acts as a competitive moat. If you are using a foundation model (like ChatGPT, GPT-3, Stable Diffusion, etc) you have to figure out where else your edge is. Why? Every other competitor has access to the same models you do, so you need to find ways to be better beyond just the common utility that everyone else is using.
A good way to think about how to be better is a mental model called the Red Queen Effect. The essence of this mental model is that you must constantly be adapting or evolving in order to survive. What this means for you and your business is that in order to succeed you need to go beyond the baseline of what everyone else is doing while understanding that the baseline is continuously ratcheting upwards. You can read more about it on Wikipedia or Shane Parrish's excellent blog post about it over at Farnam Street.
So what does that mean for you and your generative AI startup? In order to compete as a generative AI company you need to do the following well:
Data. You need to have access to data that others don't.
UI. Your UI needs to be more seamless and empowering than competitors.
Verification. You need a way to verify that the results you generate are legitimate.
Let's break those down and why they are important.
Data
Want to know what companies think is a competitive advantage? Look at what they release and don't release to the public. There are tons of open source algorithms from big tech but almost no open source data. Google doesn't release their search data, Meta doesn't release their consumer data, OpenAI doesn't release their training set for ChatGPT. Data is the competitive advantage.
Why? You've heard the term "garbage in, garbage out". The algorithms that create a model are only as good as the data being used to supply them. So when everyone is using the same models that were trained on the same data to generate outputs, you have to ask how can you make a better prediction/inference/generator than anyone else. You need to find a data advantage that you can use to either tweak the underlying foundational model or steer it towards better answers.
Generally, these foundation models are trained on the largest corpus of information that can be found, which tends to be everything available on the internet. That means typical responses are going to trend towards what an average person says or thinks on the internet. These people are not experts. If you are trying to create an expert system that requires factual information you need to have data that’s better than average. Use cases here might include things such as correctly writing code, making a medical diagnosis, getting historical facts correct, or painting the right person in an image. Improving the outputs of your model will result in a higher level of customer trust and satisfaction.
UI
Let's say you aren't able to get better data or that having better data isn't much of an improvement from what a foundation model can provide. That means you are stuck with a distribution issue if everyone is using the same underlying models. How are you going to attract users? It will be with a better user interface (UI). Why? Generative AI provides a productivity jump that's measured in orders of magnitude, which means the way people work is going to change. You need to empower people to work better in a way that both brings joy and is effortless to use.
When designing a UI for interacting with a generative AI model you have a few decisions you need to make that are intertwined with each other:
Will you generate a single artifact (text, image, video, etc) and iterate or will you generate many artifacts?
Do users need workshop tools such as tracking history, lineage, choice, and iterations?
Are you looking to augment a human or are you looking to automate a process?
Single vs Many
Depending on your use case you will want to generate a single high quality artifact such as a movie script, virtual avatar, food recipe, or piece of code or you will want to create thousands or millions of artifacts such as a mosaic of travel photos, an array of animal sounds for your game environment, or an entire crowd of virtual characters. In the first use case, you need to create an interface that enables a user to continuously refine their artifact while the second requires being able to assess and tweak results en masse without spending a copious amount of time editing each individual creation.
Workshop Tools
Users need a way to assess, tweak, and approve the results that come out of your product. This might mean having a combination of simple and advanced UIs. It might require having a high quality way to branch different versions of history and show lineage so that elements of a generated artifact can be linked together and modified. How can you show what tweaks have been made at each step without being overwhelming? For a single generation use case this might trend towards a combination of a git and Photoshop type interface.
If your use case is generating many artifacts then you need to really think about how to modify examples en masse along with displaying how changes are affecting the examples. For example if you want to change the mood from happy to ecstatic for 1,000 characters, what would that look like? How could a person make that change quickly and confirm that's the change they wanted to make? You should look to other fields that already have to do this such as sound engineers or large scale data analysis.
Augmentation vs Automation
Are you looking to empower people to do their work better and faster, or are you automating a process while trying to minimize human intervention. These are drastically different use cases that have big implications for how your UI should work. If you are augmenting the user, your metrics need to be focused on decreasing time to completion and elements that create a superior artifact. The more actions you can enable with a fewer number of course corrections, the better off you will be. In the case of automation you need ways to establish trust with the user in the fewest number of touch points possible. You will also need a lot more ways to verify the outputs at scale. Which leads us to:
Verification
You've found better data helps your product and you have a slick UI, customers should come running, right? Wrong. You're generating all of these artifacts but how do you verify that the output created is accurate and of quality? Customers are not going to trust a system beyond its use for toy problems if they cannot trust the outputs will be correct. This is a real issue, especially when you have to do it at scale. StackOverflow has temporarily banned outputs from ChatGPT due to the amount of incorrect answers it receives on the platform. They could presumably check every answer by running each question through ChatGPT and comparing the outputs. Conversely, Adobe is currently allowing generated images on its stock images site but caveats need to be included such as photorealistic images being tagged as illustrations. This it to alert people that the images contained were not made by a person.
Verification is a tricky, thorny problem. How can you determine if something is true and accurate if you haven't seen it before? This can become deeply philosophical around what it means to learn and acquire knowledge. Or you can realize that people have been making progress on the similar problem of spam filters. Luckily people have worked intensely for decades to create classification algorithms that determine the difference between different sets of items. There's a few types of verification that might be required based on your use case:
Factual Accuracy. If you are creating code or making a medical diagnosis, you need the generated text to be accurate in order to prevent harm. Depending on the type of problem you have, you may need a separate verification system that can search the required knowledge to confirm or you might be able to get away with a classification model or quick lookup. At present, the foundation models have been trained on such a large corpus of data without a bias towards expert answers that they should not be trusted for factual information for critical decisions.
Quality. If you are generating text it should have good grammar, or if you are generating images there should not be mishaps such as blurs over faces or extra fingers on a person. This is less of an issue for single artifact generation since a user can keep iterating and it is more of an issue for large scale generation where it can be time consuming to review all results manually.
On brand. If you are generating images/text/video for a customer, while checks may pass (1) and (2), you may have generated artifacts that are off brand such as language that is too formal or edgy images for a classy brand. You might be able to solve this by seeding data from a questionnaire or previous examples from a customer about what their brand is. However it is likely easier to have a tiered approval and rating system for examples that are generated.
Proper Response. If users ask for a picture of a "cute panda balancing on a beach ball" are they actually getting that image or is it off? This comes down to good prompt engineering, but you want to validate that users are getting the proper or expected response from the model instead of iterating multiple times before eventually giving up.
What all this means is that you likely need to be running additional classification models (simplest way) on top of the outputs for each of the above points that are relevant to your use case. A better way, but slower and more costly, would be to modify the underlying foundation model to account for the necessary verification steps. Alternatively, you will need some human-in-the-loop aspects to your product in order to provide sign off, establish trust, and collect training data for improving future user experience. Ultimately, all of these require high quality system design to verify artifacts at scale.
Generative AI is some amazing technology and it is finally reaching a point where a lot of people are getting excited about how the world is about to change. The pace of progress is exploding which is both exhilarating and scary. While everyone is focused on this moment of brilliance, hopefully I've given you a path for how to succeed in the coming tsunami. Focus on data, user interfaces, and verification.
SIR I have recommended your newsletter to my subscribers can you also recomend my newsletter to your subscribers so we can both grow each other
Agree that for some time, the models refined with Human in the Loop will evolve more slowly, but with a precision worth it in the long run.